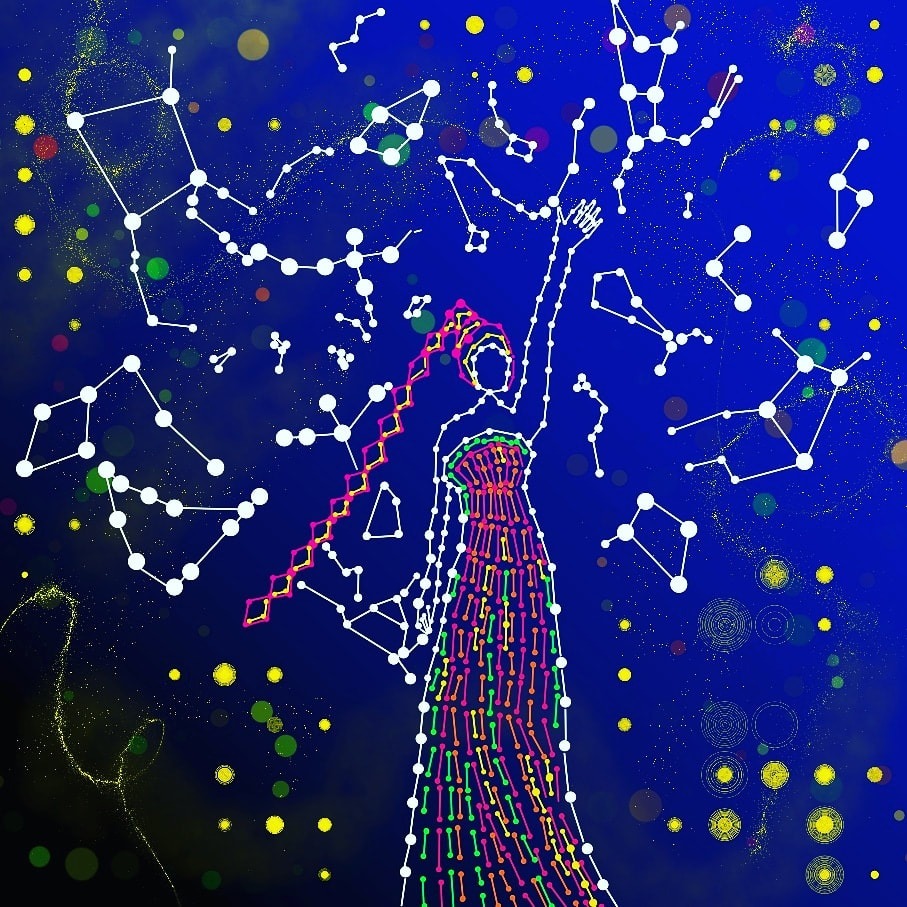
The connections between different people on a social network, the architecture behind many artificial intelligences, the ways in which different proteins interact with each other within the cell: all these seemingly very different systems can be studied by schematising them with the same type of structure, i.e. the graph. Graph representation is based on the direct interaction between pairs of agents in a system, called 'nodes'. Two friends in contact on a social network, two neurons exchanging information in a biological or artificial neural network, or two proteins sharing a certain gene expression can be schematised as two nodes joined together by a direct relationship, called an 'arc'.
The hypergraph is a natural extension of this description, in which not only pairwise interactions are considered but also (simultaneous) interactions between three or more agents. In this way it is possible to include high-order interactions in the models, i.e. involving more than two nodes at a time, which are normally ignored for simplicity's sake, but which contribute to a more accurate description of the processes.
Two new articles by the research unit Networks, one published in Nature Physics and entitled "Higher-order Laplacian Renormalisation", and one published in Nature Reviews Physics and entitled "Network Renormalisation", discuss new methods for simplifying and scaling graphs and hypergraphs, with possible applications in all fields that use this tool, from the aforementioned social networks to the neural networks of artificial intelligence and cell biology.
To understand this result, it is necessary to take a step back, starting with the concept of renormalisation mentioned in the titles of both papers. More appropriately, one should actually speak of renormalisation groupa technique that comes from theoretical physics. "The renormalisation group is a very old story in modern physics" confirm Tommaso Gili e Diego Garlaschelli, respective corresponding authors of the papers just published. Basically, the concept of renormalisation is the idea that certain characteristics of a physical system, such as a set of interacting particles or a material undergoing a phase transition, are scalableand that a simpler version can then be studied to understand the properties of the original system.
Simpler versions
Taking the example of interacting elementary particles, "under certain conditions it is possible," explain Gili and Garlaschelli, "to imagine grouping them into macro-structures, going, for example, from a thousand to a hundred particles, in order to rescale the system". This process, "one of the greatest achievements of modern physics", provides a basic set of regularity conditions, an ordered nature with a clear co-ordinate system. Unfortunately, this is not always true, and thus makes it difficult to extend the concept of renormalisation to disordered systems, characterised by elements that cannot be described by a regular, geometric space, but are rather described by a graph, precisely, through their relationships.
"Several avenues have been pursued to try to generalise the applicability of the renormalisation concept to disordered systems as well," explains Garlaschelli, director of the Networks research unit at the IMT School, "and two of the approaches that have proved most successful have been developed, independently of each other, right here in our research unit.
One of these approaches, discussed along with the other methods in the paper published in Nature Reviews Physics and proposed in a previous article, sees networks as objects intrinsically characterised by statistical fluctuations, which is then the idea behind all probabilistic mathematical models. "When the nodes of a network are aggregated producing smaller and different versions of the same network, any probabilistic graph model inevitably turns into another probabilistic model, in general different from the first," explains Garlaschelli. "So we asked ourselves: which of the two models is the correct one, since they both want to describe the same system, at different levels of resolution? We then took a radical approach: we looked for a probabilistic graph model that did not change as the scale of observation changed, i.e. that would turn into itself after rescaling the network. And we found it."
Theory advances
The model thus identified is also the only one of its kind, and in the language of statistical physics represents a so-called 'fixed point' (i.e. an element that transforms into itself) of the renormalisation group. This model constitutes not only a theoretical advance, but also a new method for analysing networks in the absence of complete information. For example, knowing the structure of inter-firm networks is crucial for correctly estimating the risk of economic stress propagation in the production system. Yet, for reasons of confidentiality these networks are usually only observable at very aggregate scales, i.e. between industries or even entire sectors. Using the 'fixed point' model found by Garlaschelli and co-workers, it is possible to 'reverse course' and move from the aggregated network to a probabilistic set of disaggregated networks consistent with it. On these disaggregated networks, it is then possible to study the propagation of stress and information, and make much more accurate predictions than those obtained from the aggregated network.
Even for the second approach, described in the paper published in Nature Physics, one has to start from previous work: 'Over the years, we have been trying to find the renormalisation group by studying the dynamics with which information travels and spreads through the various nodes of the network,' explains Gili. "Two years ago, we found". The technique discovered at the time in fact studies the so-called 'Laplacian operator', which mathematically describes precisely the dynamics of diffusion: 'You can imagine this disordered system, this network, as a series of sources, the nodes, with connections that are like pipes, through which a fluid flows. By starting the diffusion of this fluid from all the nodes of the network, you go and see how it diffuses'.
Visualising this dynamic makes it possible to understand the properties of the graph that do not change even by scaling it up, even by grouping nodes together, to obtain a graph that is therefore less complex, easier to understand and study, with the same overall properties as the original graph.
From graphs to hypergraphs
The new work starts from this solution and extends it to hypergraphs, which as we have seen, unlike normal graphs, involve arcs not only between two nodes, but also simultaneously between three, four or more nodes. "The closed two-way interaction is a segment, a edge with two nodes; that at three is a triangle; at four, with a fourth node, you have a tetrahedron; and it can grow infinitely."
The insight that led to the new result concerns the possibility of taking a hypergraph and treating the higher orders as if it were a 'simple' graph, and then applying the method found in the previous paper. "We came up with this tool," explains Gili, "which is called the Laplacian cross-orderwhich allows the process to be described by imagining that it is always a node-like structure and edge". If, for example, I have a four-node interaction, which can be visualised graphically as a tetrahedron, it can be transformed into a new graph of 'simple' nodes and edges, where the nodes represent the triangular faces of the tetrahedron and the edge the connections between the faces with one side in common.
The renormalisation processes described in the two articles make it possible to identify the possible presence, at various orders, of a scale invariance. "Scale invariance is important because, when creating a simplified representation of a structure with a certain topology (coarse graining in technical terms), the structure remains the same. Scale invariance is related to properties of interactions that are very particular and different from what is observed in regular, homogeneous systems,' Gili and Garlaschelli explain.
From biology to artificial intelligence
In addition to the case of social and economic systems, these studies see possible applications in areas such as theartificial intelligence and the biology. In the first case, the focus is on neural networks trained for Deep Learning, used for example for image recognition. "With a network with thousands of nodes at each layer, it is possible to imagine rescaling, i.e. grouping the nodes together, and see if there is a scale of renormalisation to which one can descend, to reduce the computational effort required to use the network, while still obtaining the same result.
Another possible application case is, in biology, the network of protein interactions within a cell (Protein Interaction Networks). "The interaction between proteins can be represented as a network, in which groupings can be created to be rescaled on the basis of various characteristics, for example proteins and genes that perform a similar function to each other. For example, in a bacterium such as Escherichia coli, one can obtain a structure that can in turn be subdivided into substructures in which proteins have a related task, such as energy transport and oxygen exchange'. All very current and concrete ways of using mathematical tools with an ancient history.
Jasmine Natalini